Table of Contents
Artificial Intelligence (AI) is rapidly transforming industries across the globe, offering unprecedented opportunities for innovation and efficiency. Yet, as AI’s influence expands, concerns surrounding AI ethics and bias in AI systems have grown increasingly prominent. The potentialand for AI to perpetuate or even exacerbate existing biases has sparked important discussions about fairness, transparency, and accountability in AI systems. This article delves into the intricacies of bias in AI systems, exploring the underlying causes, implications, and strategies to ensure that AI systems operate fairly and ethically.
1. Understanding Algorithmic Bias
Bias in AI systems occurs when an AI system produces outcomes that favor certain groups over others, leading to unfair or discriminatory results. This bias can stem from various sources, including biased training data, flawed algorithm design, and human prejudices. As AI systems are increasingly deployed in critical decision-making areas such as hiring, lending, and law enforcement, the potential impact of algorithmic bias becomes even more significant.
Biased Training Data
AI systems learn by analyzing historical data. If this data is biased, the AI is likely to replicate and even reinforce those biases. For example, a hiring algorithm trained on data where certain groups are underrepresented or consistently undervalued may continue to favor candidates who fit the existing, biased profile. This not only perpetuates existing inequalities but also undermines the potential of AI to drive fairer outcomes. Understanding the role of biased training data is crucial to addressing the root causes of bias in AI systems.
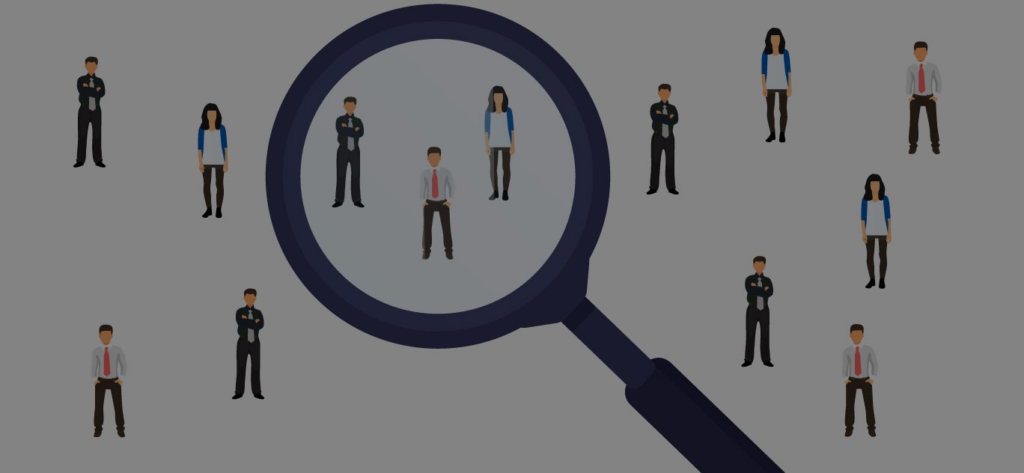
Flawed Algorithm Design
The design of algorithms plays a crucial role in shaping their outputs. Unintentionally, developers may introduce bias into AI systems through the selection of features or the weighting of different data points. Without careful oversight, these design choices can lead to skewed results that unfairly disadvantage certain groups. Addressing these flaws requires a deep understanding of the potential biases embedded in algorithmic design and a commitment to ethical AI development. Flawed design can be a significant source of bias in AI systems, making it essential to evaluate and refine these algorithms regularly.
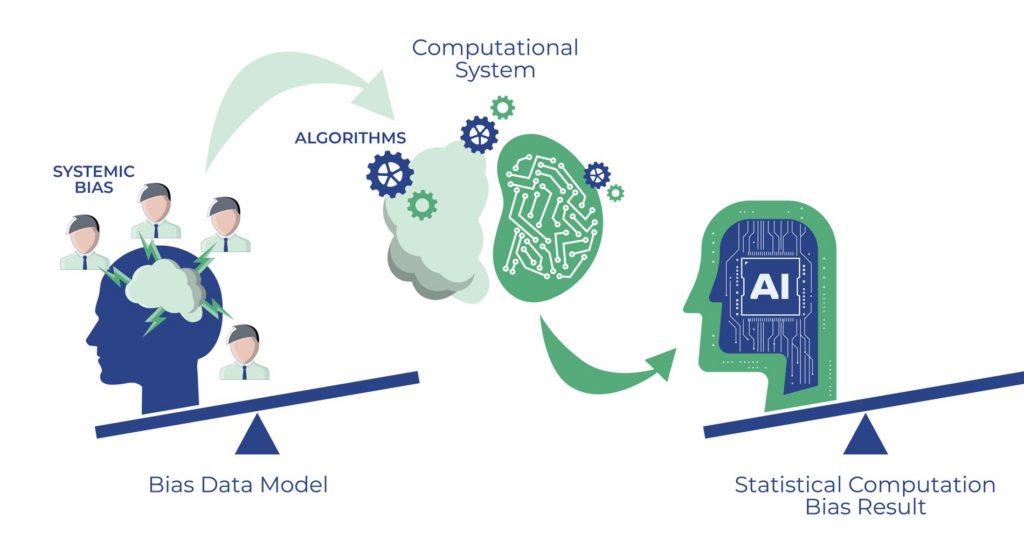
Human Biases
Human involvement in AI system development is inevitable, and with it comes the risk of introducing personal or cultural biases. From the data collection process to the interpretation of AI outputs, human decisions can reflect unconscious biases that then become embedded in AI models. This underscores the importance of diverse development teams and the need for continuous evaluation of AI systems to identify and mitigate bias. Bias in AI systems often mirrors the biases of their human creators, highlighting the need for awareness and proactive measures to prevent these biases from influencing AI-driven decisions.
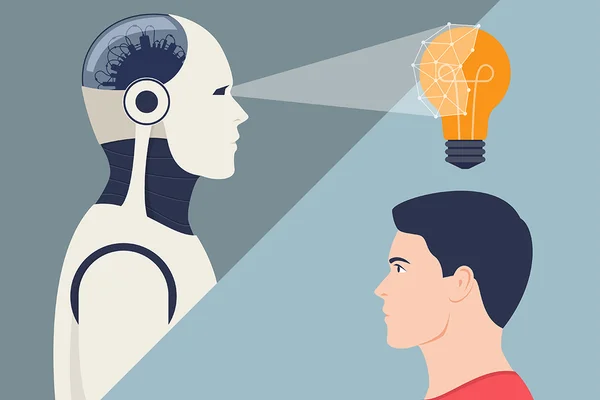
2. Implications of Algorithmic Bias
The presence of bias in AI systems can have far-reaching consequences, particularly in areas that directly affect people’s lives and livelihoods.
Discrimination
One of the most serious implications of algorithmic bias is the potential for discrimination. Bias in AI systems can lead to unfair practices in critical areas such as hiring, lending, law enforcement, healthcare, and beyond. When AI systems produce biased outcomes, they can unintentionally favor certain groups while disadvantaging others, often perpetuating or even exacerbating existing inequalities. For example, a biased AI system used in credit scoring might unfairly lower the scores of certain demographic groups based on historical data that reflects discriminatory practices, thereby restricting their access to financial services. Similarly, in hiring processes, an AI system trained on biased data might favor candidates from certain backgrounds over others, limiting opportunities for underrepresented groups.
These discriminatory outcomes harm individuals by denying them access to opportunities and resources while also eroding the credibility of AI-driven decision-making systems. When AI decisions are perceived as biased, it undermines trust in the technology and the organizations that use it, potentially leading to legal challenges and public backlash. Addressing bias in AI systems is crucial for preventing discrimination, promoting fairness, and ensuring that AI technologies contribute positively to society. By mitigating bias, organizations can create more equitable systems that support justice and equality, fostering a fairer environment for all.
Loss of Trust
Public trust in AI systems is crucial for their adoption and success. However, when bias in AI systems is evident, it can quickly erode this trust, leading to hesitation in embracing these technologies. This loss of confidence is particularly harmful in sectors like healthcare, education, and public policy, where AI could offer significant benefits. To maintain trust, it’s vital to ensure transparency in AI processes and actively address biases. Overcoming bias in AI systems is essential to building and sustaining the trust needed for AI to achieve its full potential.
Legal and Ethical Concerns
Organizations that deploy biased AI systems may face legal challenges and ethical scrutiny. As AI becomes more integral to decision-making, there is growing pressure to ensure that these systems operate fairly and transparently. Failing to address bias in AI systems not only risks legal consequences but also raises ethical concerns about the fairness and accountability of AI-driven decisions. Organizations must prioritize ethical considerations to navigate the evolving regulatory landscape and maintain their reputations.
3. Addressing Algorithmic Bias
To mitigate the risks associated with bias in AI systems, organizations can implement several key strategies designed to promote fairness and accountability in AI systems.
Diverse and Representative Data
The foundation of any fair AI system is the data on which it is trained. Using diverse and representative training data is essential to reducing the risk of replicating historical biases. By ensuring that the data reflects a broad spectrum of experiences and backgrounds, organizations can create AI systems that are more equitable and less likely to produce biased outcomes. Regularly updating and auditing datasets to reflect current realities is also crucial in maintaining fairness over time. Diverse data helps to minimize bias in AI systems and supports the development of more inclusive AI technologies.
Algorithm Audits
Regular audits of AI algorithms are an effective way to identify and address potential biases. These audits should be conducted by diverse teams that bring different perspectives to the evaluation process. By examining the outputs of AI systems and the data they rely on, auditors can uncover hidden biases and recommend adjustments to improve fairness. Algorithm audits not only enhance the quality of AI systems but also demonstrate a commitment to ethical AI practices. Regular audits are essential for detecting and mitigating bias in AI systems before they cause harm.
Bias Mitigation Techniques
Several technical approaches can help mitigate bias in AI systems. These include reweighting data points to ensure fair representation, applying fairness constraints during model training, and adjusting biased outcomes through post-processing techniques. By integrating these bias mitigation techniques into the AI development process, organizations can reduce the likelihood of biased outputs and improve the overall fairness of their AI systems. Effective bias mitigation requires a proactive approach to identifying and addressing potential sources of bias.
Transparency and Accountability
Transparency is critical in building trust and ensuring ethical AI practices. Organizations should document their AI development processes, provide clear explanations for AI decisions, and establish accountability frameworks to ensure ethical conduct. By being open about how AI systems are designed and how decisions are made, organizations can foster greater trust among users and stakeholders. Transparency also helps identify areas where improvements can be made, leading to more robust and fair AI systems. Addressing bias in AI systems requires not only technical solutions but also a commitment to transparency and accountability.
Regulation and Standards
Governments and regulatory bodies play a key role in shaping the ethical landscape of AI development. Developing and enforcing standards for ethical AI practices, including guidelines for fair data usage, transparency requirements, and accountability measures, is essential. These regulations help ensure that AI systems operate within ethical boundaries and provide a framework for addressing violations. As AI continues to evolve, regulatory oversight will be crucial in maintaining public trust and ensuring that AI technologies are used responsibly. Effective regulation is necessary to prevent and address bias in AI systems.
4. Future Directions
The future of AI ethics and algorithmic bias will involve sustained and evolving efforts to create more robust, fair, and equitable systems. As AI technology continues to advance at a rapid pace, the challenges associated with bias in AI systems will require ongoing attention and innovative solutions. Researchers and practitioners are expected to focus on developing and refining new techniques for detecting, mitigating, and ultimately eliminating bias in AI models and decision-making processes.
Interdisciplinary collaboration will play a critical role in these efforts. Addressing the complex challenges of bias in AI systems necessitates the involvement of diverse experts from various fields, including computer science, law, sociology, psychology, and ethics. This collaboration is essential because the impact of bias in AI transcends technical issues, affecting legal frameworks, social justice, and ethical standards. By bringing together different perspectives and areas of expertise, the AI community can tackle bias more comprehensively, ensuring that the solutions are not only technically sound but also socially responsible and legally compliant.
Moreover, as AI systems become increasingly integrated into everyday life, there will be a growing need to prioritize fairness, transparency, and accountability in their development and deployment. This will likely involve the establishment of new industry standards and regulatory frameworks designed to guide the ethical use of AI and prevent bias in AI systems. These frameworks will need to be adaptable, as the rapid evolution of AI technology will continuously present new challenges and ethical considerations.
Educational initiatives will also be crucial in the future direction of AI ethics. As more professionals enter the field, it will be important to equip them with the knowledge and tools to recognize and address bias in AI systems. This will involve incorporating ethics and bias mitigation strategies into AI and machine learning curricula, as well as offering ongoing training for those already working in the industry.
By prioritizing fairness and accountability, and fostering interdisciplinary collaboration, the AI community can ensure that these powerful technologies are used to benefit all members of society. The future of AI holds immense potential, but realizing this potential will depend on the collective efforts to address and overcome the challenges posed by bias in AI systems.
Conclusion
Algorithmic bias in AI decision-making poses significant challenges. However, with careful attention to data quality, algorithm design, and ethical standards, these issues can be addressed. Implementing strategies to mitigate bias and ensuring transparency and accountability can build AI systems that are fair, ethical, and trusted. As we continue exploring AI’s potential, prioritizing ethical considerations will be key to realizing its benefits while minimizing its risks.
Further Reading: For more insights into AI ethics and responsible AI development, check out our series on AI technologies and AI News.
FAQ
1. What is algorithmic bias in AI decision-making?
Algorithmic bias occurs when AI systems produce unfair outcomes by favoring certain groups over others, often due to biased training data or flawed algorithm design.
2. How does biased training data affect AI systems?
Biased training data can cause AI systems to replicate and reinforce existing biases, leading to unfair or discriminatory outcomes in areas like hiring, lending, and law enforcement.
3. What are some strategies to mitigate bias in AI systems?
Strategies include using diverse and representative data, conducting regular algorithm audits, applying bias mitigation techniques, ensuring transparency, and adhering to ethical AI development standards.
4. Why is transparency important in AI development?
Transparency builds trust by providing clear explanations of how AI systems are developed and how decisions are made, helping to identify and address potential biases.
5. What role do regulations play in addressing bias in AI systems?
Regulations establish standards for ethical AI practices, including fair data usage and accountability measures, helping to ensure that AI systems operate within ethical boundaries.
This article provides a comprehensive overview of bias in AI systems and emphasizes the importance of addressing these issues to build fair, ethical, and trusted AI systems.