Introduction
In recent years, leveraging AI for effective disaster relief has transformed how we respond to emergencies. Harnessing the power of AI, organizations now address crises with greater speed and efficiency. AI technologies are making a significant difference, from predicting natural disasters to fine-tuning resource distribution. This article explores how these advancements are reshaping disaster relief efforts and highlights key case studies that demonstrate the impactful results of this innovative technology.
1. The Transformative Role of AI in Disaster Relief
AI has profoundly transformed disaster relief efforts by offering advanced tools and capabilities that significantly enhance how emergencies are managed. Traditionally, disaster response relied on manual processes and limited data analysis. However, the advent of AI has revolutionized these methods, allowing for rapid processing of large data sets and improving overall efficiency. By leveraging AI, organizations can not only forecast disasters with greater precision but also streamline their response strategies to better meet the needs of affected communities.
AI’s integration into disaster relief has brought about several key advancements. One of the primary benefits is the ability to analyze vast amounts of data quickly. For instance, AI-driven platforms can sift through satellite imagery, social media feeds, and historical disaster data to provide real-time insights and predictions. This capability allows for more informed decision-making and strategic planning. By interpreting data from diverse sources, AI helps in identifying high-risk areas, predicting disaster impacts, and developing targeted response plans.
Predictive Analytics for Enhanced Preparedness
Predictive analytics, powered by AI, is a cornerstone of modern disaster preparedness. AI systems leverage historical data, weather patterns, and real-time information to deliver highly accurate forecasts. For example, AI models that predict hurricanes utilize satellite data and atmospheric conditions to determine the likely path and intensity of storms. This level of precision is invaluable for early warnings, enabling communities to prepare and evacuate if necessary. During Hurricane Florence in 2018, AI-driven models predicted flood zones with remarkable accuracy, which allowed for timely evacuations and efficient deployment of resources.
In addition to hurricanes, AI has been instrumental in predicting earthquakes. While earthquake prediction remains complex, AI helps by analyzing seismic data and historical patterns to identify potential risks. For example, AI algorithms can analyze minute changes in the earth’s crust to assess the likelihood of seismic activity. This proactive approach not only enhances preparedness but also improves the resilience of communities by facilitating better infrastructure planning and emergency response strategies.
Optimizing Resource Allocation
Efficient resource allocation is crucial during a disaster, and AI plays a vital role in optimizing this process. AI algorithms analyze data to determine where resources are needed most, ensuring that aid reaches the most affected areas promptly. A notable example is the 2020 Australian bushfires, where AI was used to optimize firefighting efforts. By processing real-time fire data and weather conditions, AI systems helped allocate firefighting resources to the most critical areas, significantly improving the response efforts and minimizing damage.
Another example is the use of AI in managing supply chains during crises. AI-powered systems can predict shortages and optimize logistics by analyzing data on resource availability and demand. For instance, during the COVID-19 pandemic, AI was used to manage the distribution of medical supplies, ensuring that hospitals and clinics received necessary equipment in a timely manner. This capability not only improves the efficiency of relief operations but also ensures that resources are used effectively, reducing waste and maximizing impact.
2. Case Studies Showcasing AI in Action
Real-world examples vividly illustrate how AI is reshaping disaster relief operations. These case studies highlight the transformative potential of AI technologies in improving response times, optimizing resource allocation, and enhancing overall effectiveness in crisis situations.
Hurricane Florence: AI-Powered Flood Prediction
During Hurricane Florence in 2018, AI played a crucial role in disaster management by providing accurate flood predictions. Leveraging AI for effective disaster relief involved analyzing satellite imagery, weather data, and historical flood patterns. AI systems processed these large data sets to create detailed flood maps and predict the extent of potential inundation.
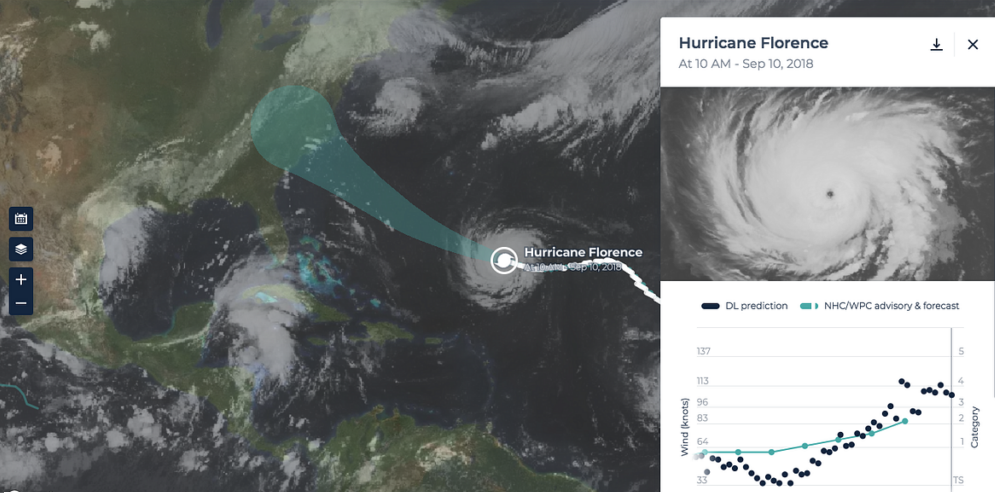
For instance, the National Oceanic and Atmospheric Administration (NOAA) used AI algorithms to enhance their flood forecasting models. These models integrated real-time weather data and satellite imagery to predict which areas were most at risk. As a result, relief organizations were able to deploy resources more strategically. They set up emergency shelters, distributed supplies, and coordinated evacuations based on the AI-generated flood predictions.
This AI-driven approach allowed for more efficient use of resources and improved the overall response to the disaster. By anticipating the areas most likely to be affected, organizations could focus their efforts where they were needed the most, ultimately reducing the impact of the flooding and ensuring that aid reached those in critical need.
Nepal Earthquake: Social Media Analysis with AI
In the aftermath of the devastating 2015 Nepal earthquake, AI demonstrated its value by analyzing social media data to assess the disaster’s impact. Following the earthquake, the sheer volume of social media posts, tweets, and messages provided a wealth of real-time information about the disaster’s effects on different regions.
AI tools were employed to sift through this massive influx of data to identify key areas requiring immediate assistance. By using natural language processing (NLP) and sentiment analysis, these AI systems could gauge the severity of the situation based on social media content. For example, AI algorithms detected mentions of urgent needs such as medical supplies and shelter, and mapped these needs against the affected regions.
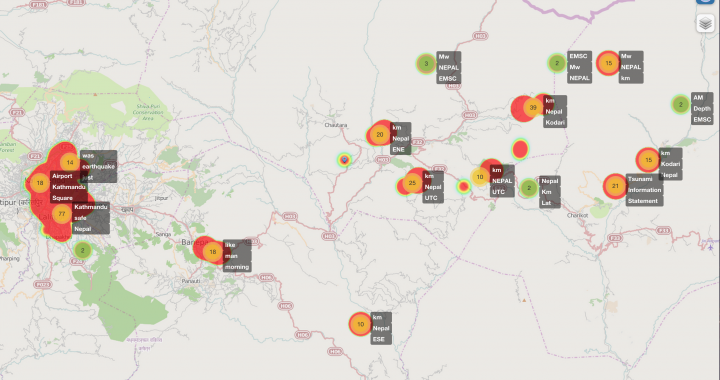
This real-time analysis provided critical insights that helped aid organizations prioritize their response efforts. They could quickly identify which communities were struggling the most and direct resources and support accordingly. The use of AI in this context not only enhanced situational awareness but also improved the speed and effectiveness of the relief efforts, demonstrating how AI can revolutionize disaster response.
3. Overcoming Challenges in AI Implementation
Leveraging AI for effective disaster relief comes with its own set of challenges, despite the considerable advantages. Understanding these challenges is crucial for maximizing the benefits of AI in managing crises. This section delves into two primary issues: data privacy and security, and the integration of AI with existing systems.
Data Privacy and Security
One of the foremost challenges in implementing AI for disaster relief is ensuring data privacy and security. Disaster relief operations often involve collecting and processing sensitive information about individuals affected by crises. This data might include personal details, health information, and location data, all of which require stringent protection.
For example, during large-scale disasters like the 2019-2020 Australian bushfires, AI was used to track and analyze data from various sources, including satellite images and social media. While this data was invaluable for planning and response, it also contained personal information that needed to be safeguarded. Ensuring that AI systems adhere to data protection regulations, such as GDPR or CCPA, is essential to maintain the confidentiality and integrity of this information.
To address these concerns, organizations must implement robust security measures. This includes encryption of data both at rest and in transit, secure authentication methods, and regular security audits. Furthermore, establishing clear data governance policies and ensuring transparency about how data is used can help build trust among the public and stakeholders. By focusing on these areas, organizations can better manage the sensitive information involved in disaster relief while leveraging AI effectively.
Integration with Existing Systems
Another significant challenge is integrating AI with existing disaster relief systems. Many organizations rely on traditional methods and legacy systems that may not seamlessly align with advanced AI technologies. This disparity can create hurdles in effectively implementing AI solutions.
For instance, during the response to the 2010 Haiti earthquake, many relief organizations were using manual data entry systems and outdated communication tools. Incorporating AI into these workflows required a careful approach to ensure that new technologies did not disrupt ongoing operations. Effective integration involves mapping out current processes, identifying potential areas of conflict, and redesigning workflows to accommodate AI tools.
An example of successful integration is the use of AI for real-time satellite imagery analysis during Hurricane Harvey in 2017. Relief organizations had to integrate AI-based image recognition systems with their existing Geographic Information Systems (GIS). This process required close collaboration between tech developers and disaster response teams to ensure that the AI tools complemented existing processes rather than causing disruptions.
Ensuring smooth integration also involves training staff and stakeholders on how to use AI tools effectively. Providing adequate support and resources during the transition phase can help mitigate resistance and ensure that the new systems enhance rather than hinder disaster relief efforts.
In summary, while leveraging AI for effective disaster relief presents challenges, addressing data privacy and security concerns, and ensuring seamless integration with existing systems are essential for overcoming these obstacles. By focusing on these areas, organizations can better harness the power of AI to improve disaster response and recovery.
4. The Future of AI in Disaster Relief
As we look ahead, the future of AI in disaster relief promises transformative advancements. With ongoing developments in technology, AI’s role in managing and responding to crises is set to become even more impactful. This section explores the potential future innovations and improvements in AI for disaster relief, focusing on how these advancements will enhance preparedness, response, and overall effectiveness.
Innovations on the Horizon
Exciting innovations are set to revolutionize how AI is utilized in disaster relief. One major area of development is the integration of real-time data from Internet of Things (IoT) devices. IoT devices, such as weather sensors, drones, and smart infrastructure, generate vast amounts of data that can be harnessed by AI systems. For example, during a natural disaster like a hurricane, IoT sensors can provide real-time updates on wind speed, rainfall, and flooding. AI can analyze this data instantaneously to provide more accurate forecasts and improve decision-making.
Another promising innovation is the enhancement of machine learning algorithms. Future AI systems are expected to feature more sophisticated algorithms that can process complex data sets with greater accuracy. For instance, machine learning models will likely become better at analyzing historical disaster data combined with real-time information to predict not only the occurrence of disasters but also their intensity and potential impact. This increased precision will enable more tailored and effective response strategies.
Moreover, AI is expected to improve in its ability to simulate disaster scenarios. Advanced predictive models will be able to create detailed simulations of various disaster scenarios, helping organizations prepare for a range of potential outcomes. For instance, AI-driven simulations could model the effects of different earthquake magnitudes on urban infrastructure, allowing disaster relief teams to plan more effective evacuation routes and resource distribution strategies.
In addition, the integration of AI with geographic information systems (GIS) will further enhance its capabilities. Improved GIS tools combined with AI can offer more detailed mapping of disaster zones and better visualizations of the affected areas. This can assist in planning and coordination efforts, ensuring that resources are allocated efficiently and that affected communities receive timely assistance.
Overall, the future of AI in disaster relief is bright with promising advancements. As AI technology continues to evolve, its applications will become increasingly sophisticated, leading to more accurate predictions, better resource optimization, and more efficient disaster responses. By staying abreast of these developments, disaster relief organizations can harness the full potential of AI to enhance their effectiveness and better serve those in need during times of crisis.
Conclusion
Leveraging AI for Effective Disaster Relief is transforming how we handle emergencies by offering advanced predictive capabilities, optimizing resource allocation, and delivering real-time insights. Case studies such as Hurricane Florence and the Nepal earthquake showcase how leveraging AI enhances disaster response and improves overall outcomes. As technology continues to evolve, the role of AI in disaster relief is expected to expand, opening up new opportunities for more effective crisis management and humanitarian aid. Embracing these advancements will lead to more efficient disaster response strategies, ultimately saving lives and minimizing the impact of emergencies.
Further Reading
To explore more about how AI is transforming various sectors, check out our series on AI news for NGOs and their applications. Learn about the latest developments and innovations that are shaping the future of technology.
FAQ
1. How does AI contribute to disaster preparedness? AI enhances disaster preparedness by analyzing data to predict potential disasters, allowing for better planning and timely response.
2. What are some examples of AI in disaster relief? Examples include AI’s role in predicting flood zones during Hurricane Florence and analyzing social media data to prioritize aid after the Nepal earthquake.
3. What challenges does AI face in disaster relief? Challenges include data privacy and security concerns, as well as difficulties integrating AI with existing systems.
4. How can AI optimize resource allocation during a disaster? AI algorithms analyze data to identify the most affected areas and predict where resources are needed most, ensuring effective deployment.
5. What future advancements are expected in AI for disaster relief? Future advancements include improved predictive models, real-time data integration, and more sophisticated machine learning techniques to enhance disaster response.