Introduction
In recent years, AI chatbot online technology has evolved from a futuristic concept to a mainstream tool, transforming the way businesses interact with their customers. With the rapid increase in online interactions, businesses require more scalable and efficient solutions to provide real-time responses, manage high volumes of customer inquiries, and maintain personalized user experiences. Traditional customer support models, while effective in the past, can no longer keep up with the demand for instant, round-the-clock service. The development of AI chatbots has solved many of these challenges, offering an automated, scalable solution that enhances customer interaction across industries.
The purpose of this article is to explore how AI chatbot online technology was developed, the technical challenges it solved, and the key components that power these chatbots. We will also examine five leading AI chatbot solutions currently dominating the market, such as ChatGPT, IBM Watson Assistant, Google Dialogflow, Microsoft Azure Bot Service, and Amazon Lex. Each of these solutions offers unique capabilities that have revolutionized customer service, personal interaction, and business automation.
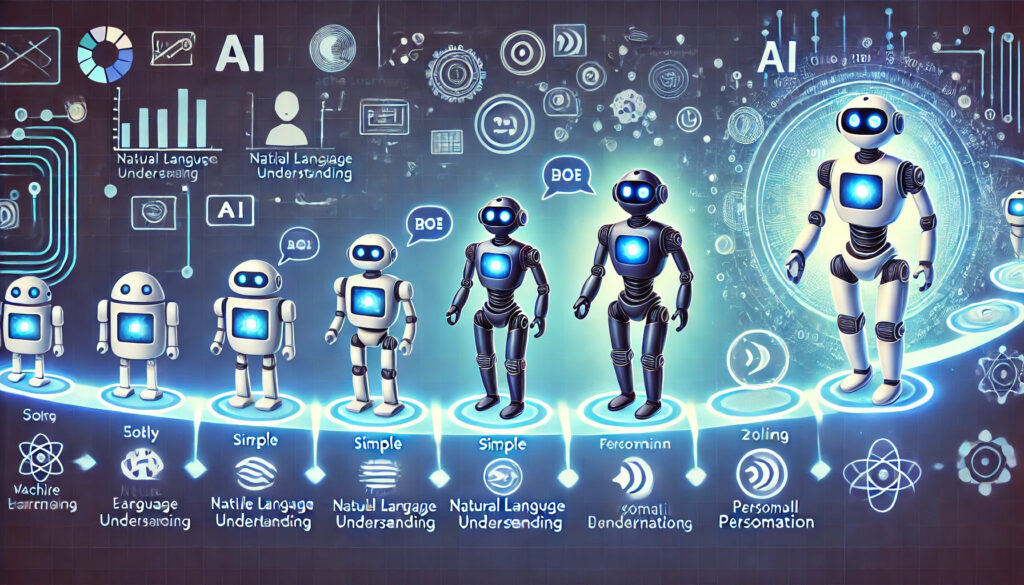
Addressing the Need for Efficient Communication
Demand for Instant Interaction
The rise of online businesses has led to a significant increase in customer expectations regarding response times. Consumers today demand instant communication, particularly when they have questions or issues with products and services. Traditional support models, dependent on human agents, often result in long wait times and inconsistent responses. This is where AI chatbots come into play. By automating responses and using natural language processing (NLP), AI chatbot online systems provide real-time responses to customer inquiries, regardless of time zones or the volume of interactions.
Managing Increased Online Traffic
As more businesses move online, the volume of customer interactions has skyrocketed, and many businesses have struggled to keep up with these demands using traditional human-only customer service models. AI chatbots are designed to handle multiple inquiries simultaneously, providing efficient service at scale. These bots help reduce wait times, resolve simple issues quickly, and reduce the burden on human agents.
Consistency and Personalization
Another key advantage of AI chatbot online technology is its ability to ensure consistency. While human agents can sometimes provide inconsistent responses due to varying levels of experience or understanding, AI chatbots rely on structured databases, ensuring that customers receive the same answers to similar questions. Furthermore, these bots offer personalized interactions by using machine learning algorithms to analyze customer behavior and preferences, delivering tailored recommendations and responses based on each user’s history.
Past Research and Development Efforts
Early AI Chatbot Models
The concept of AI chatbots dates back to the 1960s, when Eliza, one of the first rule-based chatbots, was created by Joseph Weizenbaum. Eliza used pattern matching to simulate conversation but was limited to scripted responses and lacked true conversational depth. Decades later, ALICE (Artificial Linguistic Internet Computer Entity) emerged in the 1990s, further advancing chatbot capabilities with the use of natural language processing techniques. While still relying heavily on pre-written scripts, ALICE was a significant step toward more dynamic chatbot interactions.
Natural Language Processing Progress
The development of natural language processing (NLP) played a pivotal role in the evolution of AI chatbots. In the early days, chatbots were unable to understand the context of conversations or handle complex language patterns. However, advancements in NLP in the 2000s, particularly with the development of machine learning models like Word2Vec and BERT by Google, revolutionized the way AI chatbots processed and understood human language. These models allowed chatbots to analyze language contextually, improving the quality of responses and enabling more natural interactions.
Building Conversational Systems
As businesses began to adopt AI-powered customer support, the need for more sophisticated conversational systems grew. The challenge was to create chatbots that could handle not just predefined scenarios but also adapt to new and unexpected queries. Machine learning algorithms were introduced to enable chatbots to learn from interactions and improve over time. This marked a turning point in AI chatbot development, allowing systems to engage in more fluid, human-like conversations.
Development of the First AI Chatbot Online Solution
History of the First AI Chatbot
The development of the first AI chatbot online marked a significant milestone in automating customer service. Early AI-powered chatbots were created to handle simple tasks like answering FAQs, but as demand for more sophisticated support grew, the technology evolved. The first breakthrough in AI chatbot online systems came with the introduction of natural language processing and machine learning, which allowed chatbots to simulate more human-like conversations and handle more complex tasks.
Key Contributors and Innovators
Several key players contributed to the development of AI chatbots. Companies like OpenAI and Google have been instrumental in advancing the field of artificial intelligence. OpenAI, in particular, made headlines with the development of GPT-3 and GPT-4, large language models that set a new standard for AI-driven conversations. Google, with its BERT and Dialogflow platforms, played a major role in improving chatbot language understanding and enabling more natural interactions.
Technological Achievements
The key technological achievement of early AI chatbot systems was the integration of machine learning with NLP. This allowed chatbots to analyze customer queries in real-time, understand context, and provide relevant responses. Additionally, the ability to process large amounts of data enabled these systems to continually learn and improve their performance. Case studies from companies like Amazon and Netflix demonstrated how AI chatbots could enhance customer support and improve overall user engagement.
Components of AI Chatbot Systems
AI chatbot systems consist of several key components:
- Natural Language Processing (NLP): Enables chatbots to understand and interpret human language.
- Machine Learning (ML): Allows chatbots to learn from interactions and improve over time.
- Data Integration: Connects the chatbot to databases and CRM systems to provide personalized responses.
- User Interface: The platform through which users interact with the chatbot, such as text or voice-based interfaces.
Impact on Customer Interaction
The introduction of AI chatbot online solutions has had a transformative impact on customer interaction. By automating routine tasks, businesses have been able to reduce operational costs and improve the speed and quality of their customer support. However, challenges remain, particularly in dealing with highly complex or sensitive customer issues that still require human intervention.
ChatGPT by OpenAI
History of ChatGPT
Developed by OpenAI, ChatGPT is one of the most advanced AI chatbot online systems in use today. Based on GPT-4, the latest iteration of OpenAI’s language models, ChatGPT builds on the success of earlier models like GPT-2 and GPT-3. These models use large-scale neural networks to generate human-like text, making ChatGPT one of the most powerful tools for conversational AI.
Key Contributors
OpenAI has been the driving force behind ChatGPT’s development. The company has worked closely with Microsoft, leveraging the power of the Azure cloud platform to scale and deploy the chatbot. This collaboration has enabled ChatGPT to serve millions of users across a variety of industries, from customer service to content generation.
Facilities and Achievements
ChatGPT has been used extensively in industries such as e-commerce, education, and content creation. One of its major achievements is the ability to handle complex customer queries and provide detailed, context-aware responses. The model’s success in generating natural, coherent text has set a new standard for AI chatbots.
Structure and Components
The underlying structure of ChatGPT is based on the transformer architecture, which uses deep learning to understand and generate text. The model is trained on massive datasets, allowing it to generate contextually accurate responses. ChatGPT’s integration with NLP and ML makes it one of the most sophisticated AI chatbot online systems available today.
Assessment and Challenges
While ChatGPT has been widely adopted for its versatility and advanced capabilities, challenges remain in ensuring that the chatbot provides accurate and ethical responses. The potential for generating misinformation or inappropriate content is a concern, and ongoing improvements are needed to address these issues.
IBM Watson Assistant
History of IBM Watson
IBM Watson Assistant is a powerful AI chatbot online solution that traces its roots back to IBM Watson, the AI system that famously won the game show Jeopardy! in 2011. Originally designed for complex data analysis, IBM Watson evolved into a leading chatbot platform capable of handling customer interactions in real-time.
Key Contributors
The development of IBM Watson Assistant involved contributions from IBM Research, one of the world’s leading AI research groups. The team has continuously worked on enhancing the platform’s ability to process natural language and provide accurate, contextually relevant responses.
Facilities and Achievements
IBM Watson Assistant has been widely adopted in industries such as healthcare, finance, and retail. The platform’s ability to provide accurate responses while understanding customer intent has been a key achievement. IBM Watson is known for its enterprise-level customization, allowing businesses to tailor the chatbot to their specific needs, from personalized customer interactions to automating complex workflows.
Structure and Components
The IBM Watson Assistant is built on top of IBM’s powerful natural language understanding (NLU) engine, which allows the chatbot to comprehend customer queries and generate responses that are highly accurate. The platform also includes intent recognition and entity extraction capabilities, ensuring that each conversation is context-aware and personalized to the customer’s needs.
Assessment and Challenges
While IBM Watson Assistant excels in enterprise-level applications, some challenges remain in scaling the platform for small and medium-sized businesses. Additionally, the system’s complexity may require significant customization and training, which can be a barrier to entry for organizations without in-house AI expertise.
Google Dialogflow
History of Dialogflow
Google Dialogflow was originally launched as Api.ai in 2010 before being acquired by Google in 2016. Since then, Dialogflow has become one of the leading AI chatbot online platforms, offering both text and voice interaction capabilities. It is integrated with Google Cloud and supports over 20 languages, making it a popular choice for global businesses.
Key Contributors
Google has been a significant contributor to Dialogflow’s development, leveraging its extensive expertise in AI and natural language processing. The platform has benefited from Google’s advancements in NLP, including the development of models like BERT, which improve the chatbot’s ability to understand and process complex language queries.
Facilities and Achievements
Google Dialogflow is known for its seamless integration with voice and text platforms, including Google Assistant. The platform has been used by enterprises across various industries, including customer service, healthcare, and retail. Its ability to provide omnichannel support has been one of its key achievements, enabling businesses to interact with customers across multiple platforms in real-time.
Structure and Components
At the core of Dialogflow is Google’s AI technology, which powers the platform’s speech recognition and natural language understanding capabilities. Dialogflow uses NLP models to process and interpret customer queries, enabling the chatbot to engage in more natural and fluid conversations. It also integrates with various messaging platforms and IoT devices, making it a versatile tool for businesses.
Assessment and Challenges
Dialogflow’s versatility and support for voice interactions have made it a popular choice for businesses. However, the platform’s reliance on Google Cloud may pose challenges for organizations that require flexibility in hosting solutions. Additionally, while Dialogflow excels in voice and text recognition, some users may find the platform’s learning curve steep, especially when integrating with more complex workflows.
Microsoft Azure Bot Service
History of Azure Bot Service
Microsoft Azure Bot Service is one of the leading AI chatbot online platforms developed by Microsoft. The service integrates with Microsoft Azure AI and enables businesses to create chatbots that can scale globally. The platform has been designed to handle both simple and complex customer queries, making it suitable for a wide range of industries.
Key Contributors
Microsoft, with its vast expertise in cloud computing and AI, has been instrumental in developing the Azure Bot Service. The company’s collaboration with OpenAI has further enhanced the capabilities of the platform, allowing businesses to deploy intelligent chatbots that leverage natural language processing and machine learning technologies.
Facilities and Achievements
Azure Bot Service has been adopted by industries such as retail, healthcare, and finance for automating customer support and enhancing user engagement. The platform’s ability to seamlessly integrate with Microsoft’s other tools, such as Teams and Dynamics 365, has been a major achievement, allowing businesses to leverage the full power of Microsoft’s ecosystem.
Structure and Components
Azure Bot Service is built on Microsoft Azure’s cloud infrastructure, which allows for scalable, reliable deployment of chatbots. The platform integrates with LUIS (Language Understanding Intelligent Service), enabling the chatbots to process and understand customer queries with high accuracy. Additionally, Azure Bot Service can be integrated with voice, SMS, and messaging platforms, making it a flexible solution for businesses of all sizes.
Assessment and Challenges
While Azure Bot Service offers powerful features and seamless integration with Microsoft products, some users have reported challenges with the platform’s complexity. Businesses may require technical expertise to fully implement and customize the service, particularly when integrating it with existing enterprise systems.
Amazon Lex
History of Amazon Lex
Amazon Lex is a cloud-based AI chatbot online platform developed by Amazon Web Services (AWS). Launched in 2017, Lex is built on the same technology that powers Alexa, Amazon’s voice assistant. The platform is designed to handle both text and voice interactions, making it a popular choice for businesses looking to offer conversational AI experiences.
Key Contributors
Amazon has been at the forefront of AI innovation with its development of Lex. The team behind Amazon Alexa played a crucial role in the development of Amazon Lex, leveraging their expertise in speech recognition and natural language understanding to create a chatbot platform that excels in both voice and text-based interactions.
Facilities and Achievements
Amazon Lex has been adopted by companies in industries such as e-commerce, customer service, and healthcare. Its ability to integrate with AWS Lambda allows businesses to create highly customized conversational workflows. The platform’s real-time speech recognition capabilities have also made it a favorite for businesses looking to automate voice-based customer support.
Structure and Components
The core components of Amazon Lex include automatic speech recognition (ASR) and natural language understanding (NLU), which enable the platform to process and respond to customer queries in real-time. Amazon Lex integrates with other AWS services, such as Amazon S3 and Amazon DynamoDB, to deliver a scalable, secure chatbot solution. Additionally, Lex supports multi-turn conversations, allowing it to handle more complex customer interactions.
Assessment and Challenges
While Amazon Lex is a powerful tool for businesses looking to automate voice and text interactions, the platform’s reliance on AWS services may be a limitation for organizations that prefer a multi-cloud approach. Additionally, some businesses may find the platform’s integration with existing systems to be complex, requiring a certain level of technical expertise to fully implement.
Conclusion
In summary, AI chatbot online technology has revolutionized how businesses interact with customers, providing scalable, efficient, and personalized support. Through the development of advanced systems like ChatGPT, IBM Watson Assistant, Google Dialogflow, Microsoft Azure Bot Service, and Amazon Lex, the customer service industry has been transformed. These solutions have significantly improved customer engagement, reduced operational costs, and set new standards for real-time, personalized communication. However, challenges remain in ensuring accuracy, ethical use, and handling complex queries.